The Link Between AI, Electronics, And Sustainability
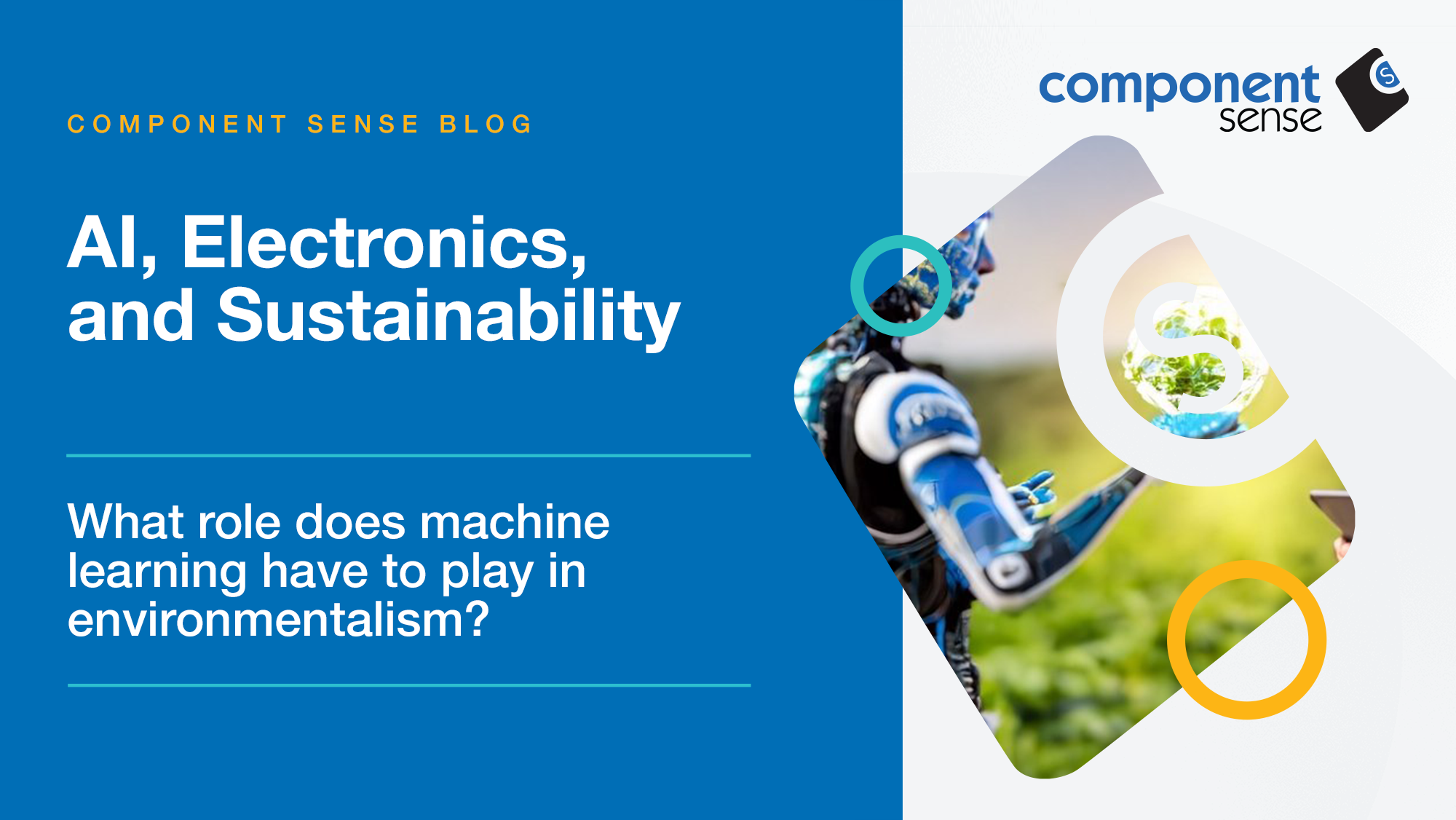
By the end of 2022, a revolutionary technical marvel seemed unleashed on society.
However, the story of artificial intelligence (AI) dates back to 1952, when computer scientist Arthur Samuel created software to play checkers. Today, AI is reshaping industries across the globe, including businesses' approach to sustainability.
Research by PwC models the impact of AI on managing agriculture, water, energy, and transport. It estimates that machine-learning environmental applications could contribute $5.2 trillion to the global economy in 2030. However, the production of AI components and the training of AI models also deplete resources and contribute to pollution at concerning levels.
Component Sense is proud to champion the UN sustainability goals. As a business, we are excited by the possibilities of machine-learning but also cautious of its potential for environmental damage. As you read this blog, try to guess which images were generated by AI!
Negative impacts of AI on the environment
“Think of it from a fundamental point of view. AI is just applied statistics. If you want it to work, you need good data from existing sources. If you have bias, the machine-learning algorithm will amplify this,” explains Component Sense Developer, Subhomoy Haldar.
As well as ethical dilemmas, the production of AI itself is fraught with controversies. Environmental concerns arise not only from manufacturing components but also from training large models.
The environmental footprint from training AI models
Impressive traits of machine-learning often come at the cost of polluting the atmosphere. A study by the University of Massachusetts found that training a single AI model can emit as much carbon dioxide as the lifetime of five cars. OpenAI researchers say that since 2012, the computing power needed to train cutting-edge machine-learning models has doubled every 3.4 months.
The deep learning required for advanced AI is built on layers and layers of neural networks, often with billions of parameters. Some complex AIs undergo training on multiple GPUs for weeks or even months. The training of machine-learning models takes place in industrial data centres, where copious amounts of energy, often generated by fossil fuels, are used to operate machinery and keep rooms at the desired temperature.
Thankfully, the high energy requirements for cooling data centres are not going unnoticed. Many data centre operators are looking to start operations in cool parts of the world. For example, Google is investing in data centres in Nordic countries. As well as having a cooler climate, all of Iceland’s energy comes from 100% renewable geothermal and hydroelectric power.
The environmental footprint from fabs (fabrication plants)
Fabs often create hazardous waste and require millions of litres of water to operate. The demand for new and improved AI chips is ramping up the construction of countless new fabs, multiplying environmental concerns. Some fabs are also built in locations where biodiverse ecosystems would otherwise flourish.
Thankfully, systems are in place to ensure the correct steps are taken to minimise the environmental impact of new fabs. In the USA, the National Environmental Policy Act (NEPA) forces federal agencies to review the potential ecological consequences of new fabs and inform the public. NEPA applies to many federally funded projects, including new fabs supported by the CHIPS Act.
Resource extraction
A large quantity of rare earth elements (REEs) are required to produce AI components. Although REEs are not particularly sparse, the challenge lies in their low concentrations and geothermal properties that complicate extraction. Nevertheless, REEs are finite, meaning an increase in procurement is cause for concern.
Some electronic devices contain about 60 REEs. Thankfully, machine-learning could be helping with its own production. AI-based mineral exploration algorithms are beginning to be utilised to identify geological structures and mineral deposits. Miners then use drones for autonomous drilling.
Positive impacts of AI on the environment
“The goal of AI should be to improve human life. It should be impactful for people around us and the environment. In an ideal world, we will use AI to remove injury-prone and repetitive tasks,” said Subhomoy.
When weighing up the environmental impact of machine-learning, it is vital to consider its positive attributes. Not to mention the vast opportunities that could be possible as machine-learning technology continues to advance. PwC estimates that AI could reduce greenhouse gas emissions by 4% in 2030.
Energy efficiency
Despite requiring large amounts of energy to be trained and manufactured, AI can also help businesses to be more efficient. Machine-learning can potentially offset its own energy footprint by predicting energy usage patterns and optimising consumption. Tesla uses AI driving features to optimise driving patterns and increase efficiency.
AI is also being utilised to push the boundaries of renewable energy sources, such as solar and wind. GE Renewable Energy utilises AI to enhance the performance of their wind turbines. This is done using sensors and algorithms to predict wind conditions and adjust turbines accordingly.
E-waste management
The dumping of large amounts of electronic waste (e-waste) is one of the main reasons for the inception of Component Sense. We are intrigued by how machine-learning may be able to help electronic manufacturers to reduce waste.
Companies like Recycleye are using AI-powered machinery to separate sought-after recyclable materials like REEs from waste streams. Ultimately, this helps to create a more circular electronics industry.
Disaster relief and preparedness
The link between climate change and severe weather events is well documented. AI helps analyse weather patterns and issue early warning signals before extreme weather events occur, mitigating the negative effects of climate change.
The xView2 program combines the best of computer vision and machine-learning with satellite imagery to pick out buildings damaged in natural disasters. This application from the Defence Innovation Unit in the U.S. Department of Defence could support first responders and search-and-rescue teams.
Use cases of AI for electronic manufacturers
Like in many other industries, electronic manufacturers seek ways to integrate AI into their business processes. Generally, the goal is to gain efficiencies, but the possibility of machine-learning helping businesses to operate more sustainably should not be ignored.
Production control and maintenance
As mentioned earlier, AI is only as valuable as the data that it is trained on. For electronic manufacturers, this may come as welcome news. Having a machine-learning system work in tandem with a modern and well-documented MES (manufacturing execution system) allows it to create detailed forecasts.
“In electronics, there is more information, barcodes, and labels than most other industries. So, AI can bring a very scientific approach to processes,” explains Subhomoy.
Design optimisation
Designing PCBs (printed circuit boards) has usually been a time-intensive and manual process. The reliance on people means that ideating PCBs has been prone to human error. AI can interpret enormous amounts of data. This capability means machine-learning can analyse specifications, signal requirements, thermal profiles and more.
Sustainable supply chains
As well as reducing excess, AI may allow your business to increase throughput ties and reduce bottlenecks. Machine-learning could even monitor conditions to predict maintenance on your product line.
When it comes to transporting components, AI-driven vehicles are helping to gain fuel efficiencies. This reduction in carbon footprint is due to energy-efficient vehicles and plotting the most effective route possible.
The impact of AI on the electronics industry workforce
It is an unavoidable fact that AI will displace many jobs, and the electronics industry is no different. A recent survey in the US found that 52% of adults are more concerned than excited about the role of machine-learning in daily life. On the other hand, PwC estimates that AI could create 38.2 million new jobs across the global economy.
In an ideal world, AI will free up dangerous and repetitive jobs, allowing people to add more value to their workplace. However, machine-learning will not replace every role.
“In instances when you need a 100% true answer, AI based on statistical models is insufficient. Electronic manufacturers must associate a certain level of risk with their answer. At the end of the day, a machine can not be held accountable,” said Subhomoy.
Want more advice on managing your component inventory?
AI could help electronic manufacturers prioritise sustainable business practices. Our Excess and Obsolete Experts utilise a simple and controllable algorithm to analyse lists of excess components. This way, we can find pricing and value information more quickly and accurately. A human then cross-checks all data.
The future of AI in electronics is uncertain. If the production and teaching of machine-learning are refined to reduce the environmental impact, this technology could have a large role in promoting sustainability, not just in the electronics industry.
To make your supply chain more sustainable by redistributing excess component stock, speak to a member of the Component Sense team.